How AI Is Poised to Upend Cloud Networking
To fully leverage the cloud for hosting AI workloads, optimizing your cloud networking strategy is essential.
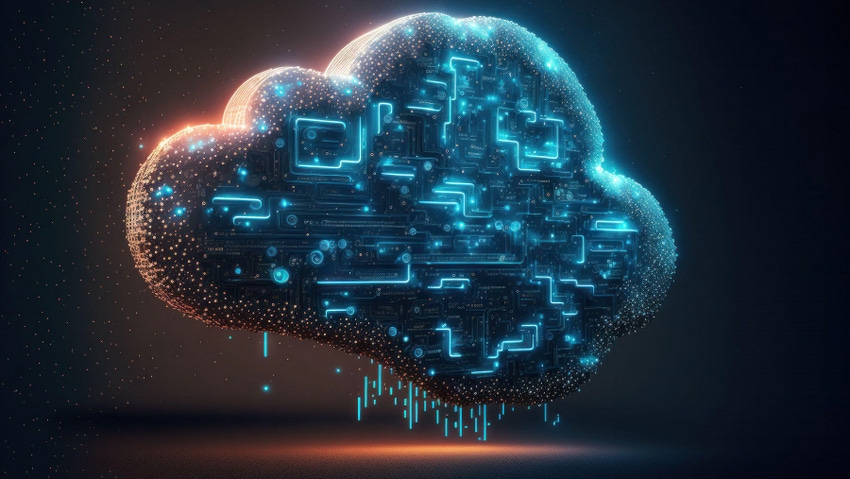
Much has been said about how AI will accelerate the growth of cloud platforms and enable a new generation of AI-powered tools for managing cloud environments.
But here's another facet of the cloud that AI is likely to upend: networking. As more and more AI workloads enter the cloud, the ability to deliver better cloud networking solutions will become a key priority.
Here's why, and what the future of cloud networking may look like in the age of AI.
AI's Impact on Cloud Networks
The reason why AI will place new demands on cloud networks is simple enough: To work well at scale, AI workloads will require unprecedented levels of performance from cloud networks.
That's because the data that AI workloads need to access will reside in many cases on remote servers located either within the same cloud platform where the workloads live or in a different cloud. (In some cases, the data could also live on-prem while the workloads reside in the cloud, or vice versa.)
Cloud networks will provide the essential link that connects AI workloads to data. The volumes of data will be vast in many cases (even training a simple AI model could require many terabytes' worth of information), and models will need to access the data at low latency rates. Thus, networks will need to be able to support very high bandwidth with very high levels of performance.
Is Cloud Networking Ready for AI?
To be sure, AI is not the only type of cloud workload that requires great network performance. The ability to deliver low-latency, high-bandwidth networking has long been important for use cases like cloud desktops and video streaming.
Cloud vendors have also long offered solutions to help meet these network performance needs. All of the major clouds provide "direct connect" networking services that can dramatically boost network speed and reliability, especially when moving data between clouds in a multicloud architecture, or between a private data center and the public cloud as part of a hybrid cloud model.
But for AI workloads with truly exceptional network performance needs, direct connect services may not suffice. Workloads may also require optimizations at the hardware level in the form of solutions such as data processing units (DPUs), which can help process network traffic hyper-efficiently. Indeed, vendors like Nvidia, which has unveiled an Ethernet platform tailored for generative AI, are already investing in this area — and it says a lot that a company mostly known for selling video cards is also recognizing that unlocking the full potential of AI requires networking hardware innovations, too.
The Future of Cloud Networking: What to Expect
For now, it remains to be seen exactly how cloud vendors, hardware vendors, and AI developers will respond to the special challenges that AI brings to the realm of cloud networking. But in general, it's likely that we'll see changes such as the following:
Greater use of direct connects: In the past, cloud direct connect services tended to be used only by large businesses with complex cloud architectures and high performance needs. But direct connects could become more commonplace among smaller organizations seeking to take full advantage of cloud-based AI workflows.
Higher egress costs: Because cloud providers usually charge "egress" fees whenever data moves out of their networks, AI workloads running in the cloud could increase the networking fees that businesses pay for egress. Going forward, the ability to predict and manage egress charges triggered by AI workloads will become an important element of cloud cost optimization.
Fluctuating network consumption: Some AI workloads will consume cloud network resources at high volumes, but only for a temporary period. They may need to move vast quantities of data while training, for example, but scale their network usage back down when training is complete. This means that the ability to accommodate wide fluctuations in network consumption is likely to become another important component of cloud network performance management.
Conclusion
There's no way around it: If you want to take full advantage of the cloud to help host AI workloads, you need to optimize your cloud networking strategy — a move that requires taking advantage of advanced networking services and hardware, while also adjusting cloud cost optimization and network performance management strategies.
For now, the solutions available to help with these goals are still evolving, but this is a space to follow closely for any business seeking to deploy AI workloads in the cloud.
About the Author(s)
You May Also Like