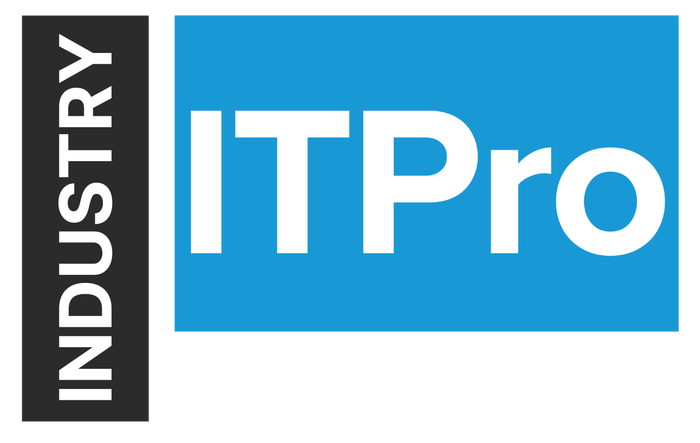
Insight and analysis on the information technology space from industry thought leaders.
Want to Become a Data Hero? Learn From DevOps
Here are three factors that will have the biggest impact on the success of your organization's data and analytics.
March 15, 2023
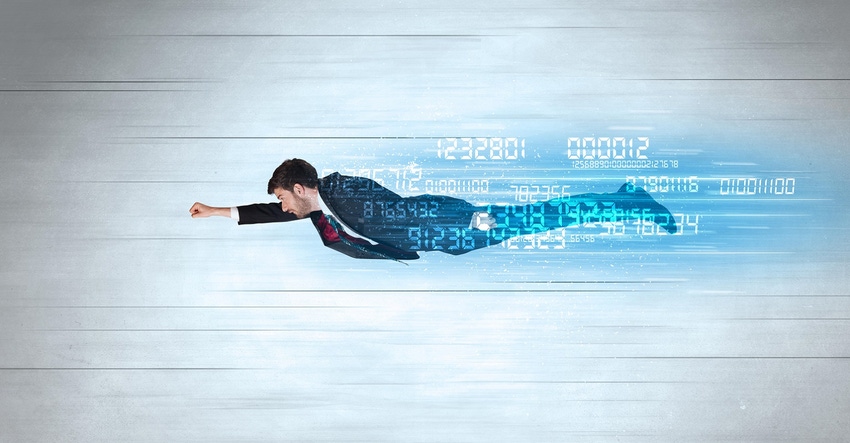
All around us in our personal lives, we see examples of the proliferation of data. Autonomous vehicles account for 1.4 terabytes of data every hour. There are, on average, 25 connected devices in every home, up from 11 in 2019.
The explosion of data is not just confined to our personal lives and business-to-consumer organizations. Across industries, the production and consumption of data is increasing at a mind-boggling rate.
But at many organizations, data is unanalyzed and unused. A large percentage of data and analytics transformations programs at many companies fail, with 46% of AI and machine learning projects never making it to production. Even when organizations have individual data success stories, they struggle to scale those competencies to other departments.
There's hope. DataOps applies the principles of agile development and DevOps to data management and analytics, improving success rates. It rapidly turns new insights into business deliverables and action plans. I've helped multiple companies implement DataOps strategies. Three factors have the biggest impact on the success of data and analytics programs:
Improve Collaboration. In large organizations, data pipeline workflows are messy, to say the least. The stakeholders span line-of-business owners, the digital teams, the analytics team, data managers and security. Within each of those teams, there are multiple personas. The complexity of these workflows can lead to decisions made with incomplete information, where one group acts without input from another key stakeholder group, leading to errors. It is useful to categorize these stakeholders into two categories. Whereas DevOps workflows improve efficiency by improving collaboration between developers and operations teams, DataOps calls for improved collaboration from two sides of the data pipelines: data suppliers and data consumers. Foster collaboration by providing a technical platform to align these two broad stakeholder groups.
Introduce Pervasive Automation. The core of DataOps is the automated data pipeline, which describes a set of functions that gather, aggregate, cleanse, perform quality-control audits, publish, deploy, and track usage of data. These functions are supported by a wide set of data infrastructure components. The right DataOps plans are built to serve multiple data consumers, from traditional data analysts who use business intelligence tools to the data scientists who use most sophisticated analytics platforms and machine learning pipelines. The backbone of data pipeline automation, in turn, is orchestration. Orchestration is an absolute must-have in order to coordinate across the discrete data management tools and functions, and ultimately automate from data source to fully operationalized insights.
Get Standardization, Investment, and Governance Right. Whereas collaboration and automation focus on the people and tools that interact directly with the data pipeline, there are strategic issues that influence the success of data and analytics pipeline as well. Broadly, these can be categorized as standardization, strategy, and process questions. The first question can be summarized as follows: Are your processes standardized to a degree that they allow you to collect meaningful data? When you use data, are you sure it was collected in a uniform manner? Second question: Is this digital and analytics plan you've developed the right fit for your organization? I know of companies that deploy data programs for specific lines of business, but only do so if the estimated return on investment is an order of magnitude greater than previous processes. And third question: Is your data governance strategy sufficiently balanced so as not to be too overly burdensome or lax in the face of government regulations? Answering these questions in a way that makes sense for your organization are important factors in the success of a data analysis project.
Just as DevOps was implemented to do away with cumbersome waterfall approaches to software development, DataOps strategies are similarly designed for continuous improvement. As such, they can represent a large shift in workflows and processes at many organizations, which may seem daunting.
That's why I recommend that most organizations start small. Take one well-defined use case and implement a DataOps workflow, with an emphasis on collaboration and automation. Apply the learnings and takeaways from that experience to subsequent efforts as you scale DataOps practices across the organization.
Which brings me to the big benefit of DataOps. At most organizations, the benefits of nearly every data analytics use case are siloed and fragmented, with competencies varying between teams and functions. Organizations that scale DataOps programs can democratize data analytics and also create a virtuous cycle of capability improvement that extends to a broader range of processes and stakeholders.
Ram Chakravarti is CTO of BMC.
About the Author(s)
You May Also Like